Deep learning-based techniques for service robots
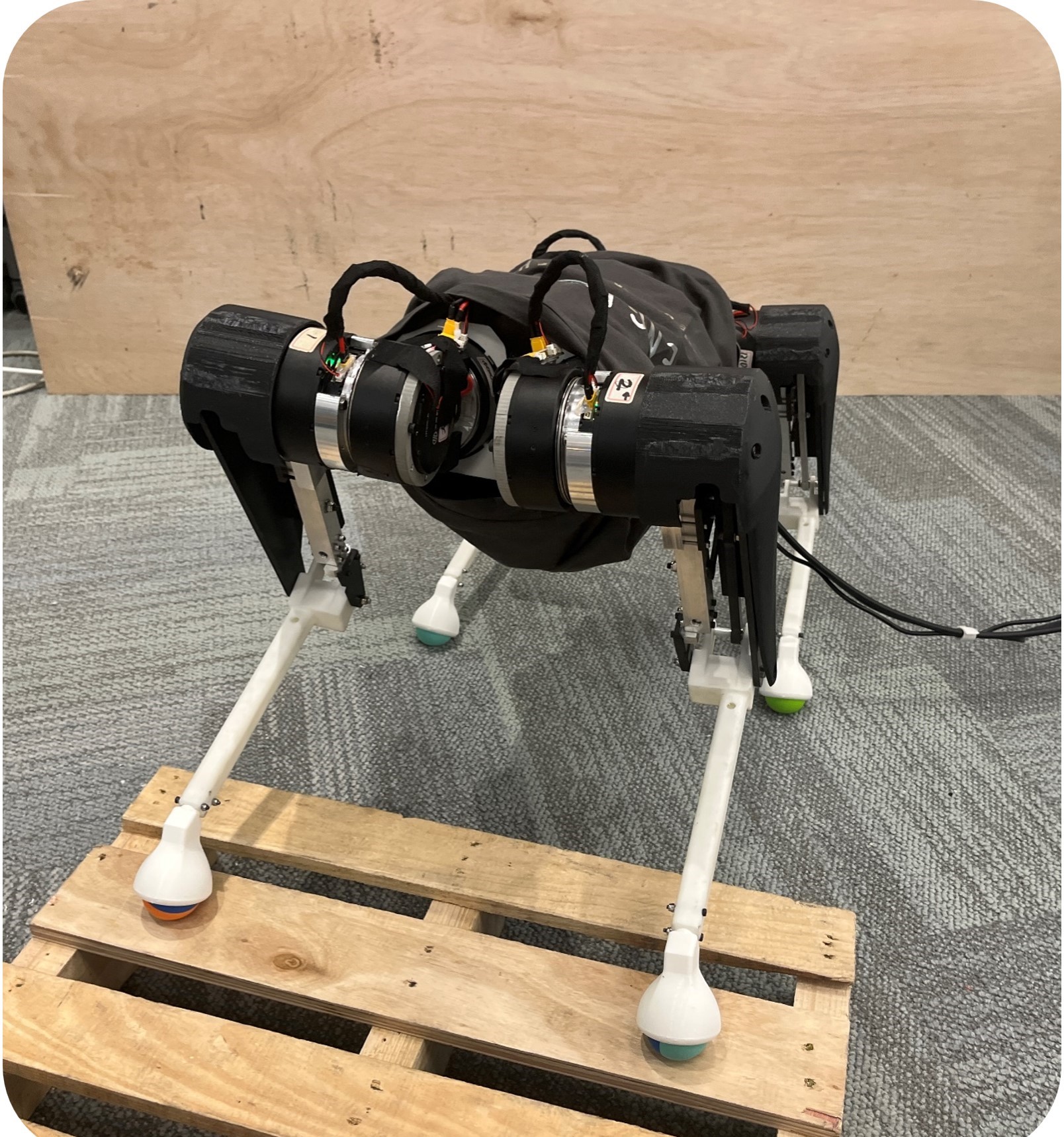
We aim to use deep learning-based techniques to enhance the intelligence of legged robots. Deep learning helps robots to better understand the environment. Deep reinforcement learning assists robots with navigating within the environment. Finally, Large Language Models allow robots to interact with the environment or humans.
Example research
Deep reinforcement learning for highly dynamic skills and challenging terrains
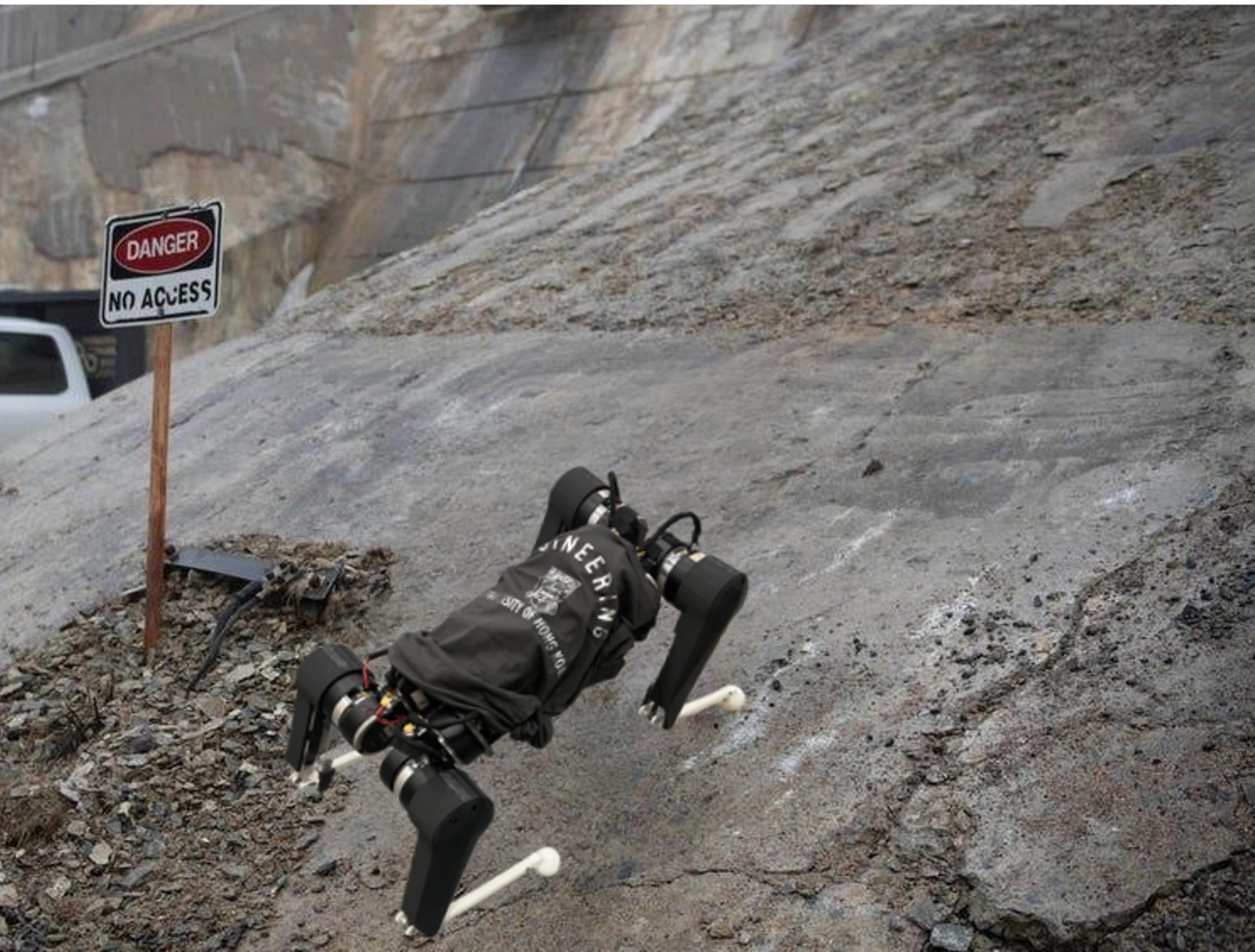
We aim to use deep reinforcement learning to enhance the performance of legged robots when operating in highly challenging terrains or environments. We also aim to use DRL to let legged robots learn highly-dynamic skills or deal with highly challenging scenarios such as walking in the presence of complete motor failures.
Example research
Autonomous pick and place with obstacle avoidance using point clouds

Main goal is to enable autonomous operation of robots using vision and control techniques. In terms of vision, depth camera is used to obtain point clouds of the environment. For control, motion planning and obstacle avoidance techniques are investigated to guarantee the successful operation of the robots.
Link to the demo
High precision control for construction and hydraulic robots
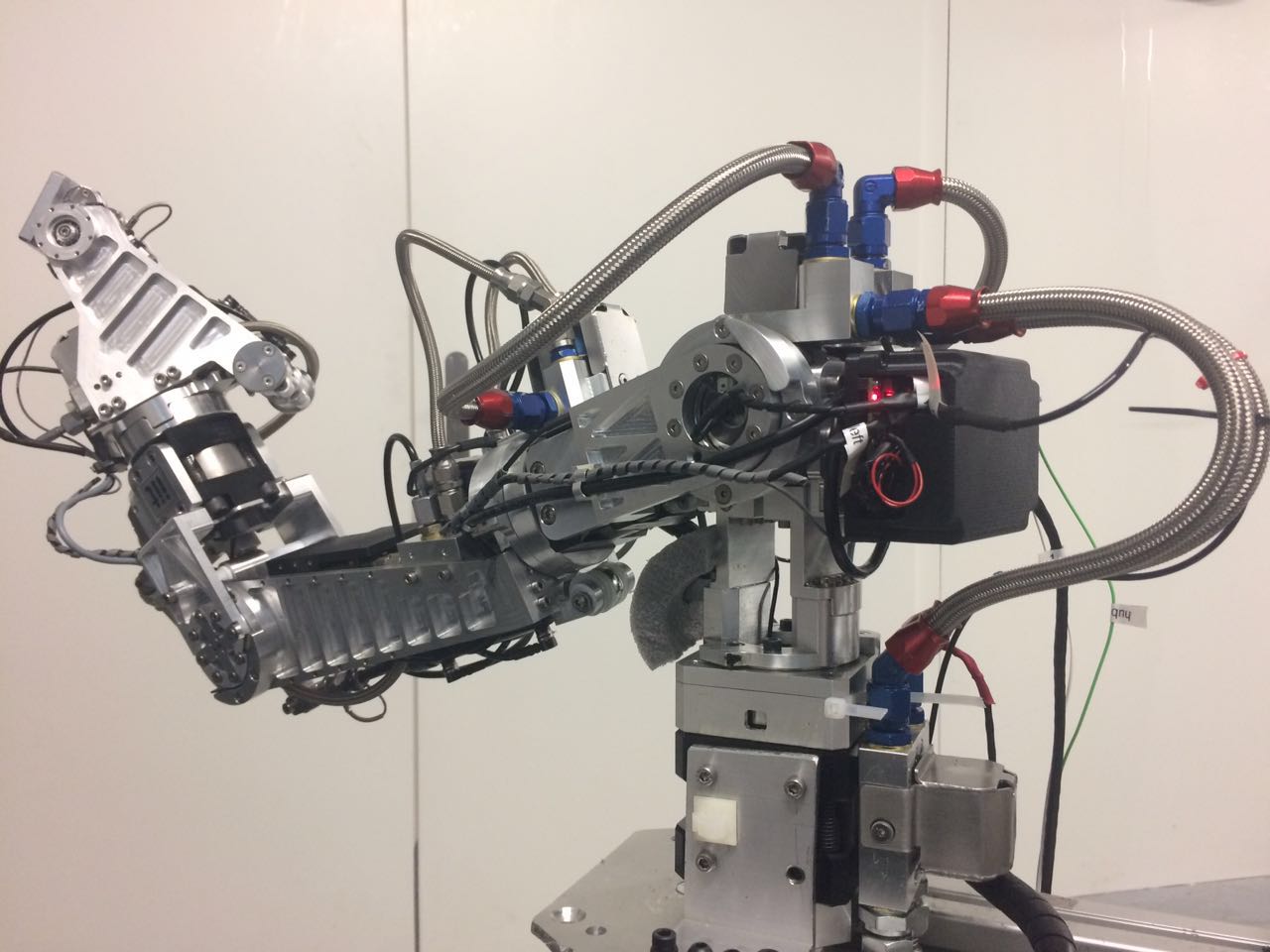
This research on this hydraulic robotic arm was performed in ADRL, ETH Zurich and supported by NCCR digital fabrication. The difficulty in controlling this robotic arm lies in the nonlinearities and significant friction forces in the hydrualic actuators. The aim of this research is to increase the tracking precision of this hydraulic robotic arm such that it can be used for tasks such as construction and manipulation tasks. Vision-based control techniques are required to obtain its position in 3D operational space.
link to NCCR digital fabrication
link to high precision control for hydraulic robots